This breakthrough technology, based on Transformer architecture, offers a solution to challenges in identifying irregular disease patterns and complex backgrounds in rice cultivation. With its promise of higher precision, AISOA-SSformer could become a valuable tool for farmers and agricultural experts, contributing to better crop health and management.
Rice is one of the world's most essential food crops, but its production is constantly threatened by leaf diseases caused by pathogens like fungi, bacteria, and viruses. These diseases, which manifest as spots or blotches on leaves, can severely impact crop health and yield. Traditional manual identification of such diseases is labor-intensive and error-prone. The advent of deep learning-based segmentation technologies has brought improvements, but current methods often struggle with irregular disease features, complex backgrounds, and blurred boundaries in leaf images.
A study (DOI:10.34133/plantphenomics.0218) published in Plant Phenomics on 5 August 2024, helps farmers make better decisions, resulting in healthier crops and higher yields while reducing environmental impact.
The AISOA-SSformer model introduces several new components to enhance performance in rice leaf disease segmentation. The team implemented the model using PyTorch 1.10.0 to ensure consistency across experiments. The Sparse Global-Update Perceptron (SGUP) is employed to stabilize the learning process, effectively capturing the irregular features of leaf diseases. Additionally, a Salient Feature Attention Mechanism (SFAM) is incorporated to help the model filter out background noise while focusing on important features. This is achieved through two key modules: the Spatial Reconstruction Module (SRM) and the Channel Reconstruction Module (CRM), which work together to separate and optimize disease features.
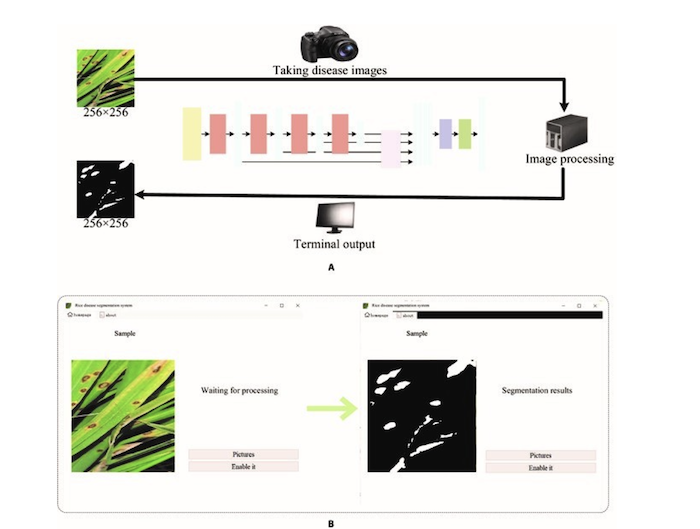
Fig.7 (A and B) Schematic diagram of the disease segmentation system and system demonstration example Source: Chinese Academy of Sciences
To further enhance accuracy, the model uses the Annealing-Integrated Sparrow Optimization Algorithm (AISOA), which adjusts the training process to avoid being trapped in local optima and improve the recognition of fuzzy leaf edges. Compared to existing models, AISOA-SSformer achieved an impressive 83.1% mean intersection over union (MIoU), an 80.3% Dice coefficient, and a recall of 76.5%, making it one of the most accurate methods for segmenting rice leaf diseases. Ablation studies confirmed the combined impact of SGUP, SFAM, and AISOA, boosting the MIoU and Dice coefficients significantly. Comparative analyses with established models, including CNN-based and Transformer architectures, highlighted AISOA-SSformer’s superior ability to segment rice leaf diseases, even in complex environments, emphasizing its potential for practical agricultural applications.
The AISOA-SSformer model represents a breakthrough in precision agriculture, providing an advanced tool for identifying rice leaf diseases. By enhancing segmentation accuracy and addressing complex backgrounds and irregular disease patterns, this model has the potential to revolutionize crop disease management. In the future, it could be applied to other crops and agricultural challenges, making significant contributions to sustainable farming and food security.